- The routing API and parameters etc are very nice to work with IMO.
- It was very easy to get json by default support setup.
- Metrics were very easy to wire up.
- Swagger integration was pretty rough, while it looks good on paper I could not get an example to show up, and it is unable to handle case classes or enums which we use.
- Benchmark performance when I’ve looked around the web was pretty bad, I’ve not done enough to figure out if this is real or not. I’ve seen first hand a lot of benchmarking are just wrong.
- Integration with JUnit has been rough and I cannot seem to get the correct port to fire, I suspect I have to stop using the @Test annotation is all (which I’m not enjoying).
- Http/2 support is still lacking despite being available in the version of Jetty they’re on, I’ve read a few places that an issue is keeping web sockets working but either way there is no official support in the project yet.
- This doesn’t distribute the data well and therefore misses the point of a distributed database.
- There are practical limits on the performance of partition sizes
- rows are read serially one node at time until limit is reached.
- a suboptimal storage layout leading to very large partitions if the data distribution of the secondary index is not ideal.
- contacts should have first name, last name, address, state/region, country, postal code
- lookup by contacts id
- retrieve all contacts by a given last name
- retrieve counts by zip code
- songs should have album, artist, name, and total likes
- The contact list exercise, can be used as a basis for the “users”, users will have no login because we’re trusting people
- retrieve all songs by artist
- retrieve all songs in an album
- retrieve individual song and how many times it’s been liked
- retrieve all liked songs for a given user
- “like” a song
- keep a count of how many times a song has been listened to by all users
- use the music service data model as a basis, we will be tracking each “registered device” that uses the music service
- a given user will have 1-5 devices
- log all songs listened to by a given device
- retrieve songs listened for a device by day
- retrieve songs listened for a device by month
- retrieve total listen time for a device by day
- retrieve total listen time for a device by month
- retrieve artists listened for a device by day
- retrieve artists listened for a device by month
- first initialize the keyspace with RF3
cassandra-stress "write cl=ONE no-warmup -col size=FIXED(15000) -schema replication(strategy=SimpleStrategy,factor=3)"
- second run stress
cassandra-stress "mixed n=1000k cl=ONE -col size=FIXED(15000)
- repeat as often as you’d like with as many clients as you want.
- use python3 and virtualenv
python -m venv venv
- source venv/bin/activate
- read and follow install docs
- if you want to skip the docs you can get away with
pip install cassandra-driver
- install a faker library
pip install Faker
- download java 8
- create a command line application in your project technology of choice (I used maven in this example for no particularly good reason)
- download a faker lib like this one and the Cassandra java driver from DataStax again using your preferred technology to do so.
- run the following code sample somewhere (set your RF and your desired queries and data model)
- use different numbers of clients at your cluster until you get enough “saturation” or the server stops responding.
- Are the nodes saturated or not? IE have you thrown enough client load at them?
- What does GC look like? Use a tool like gceasy with the logs or sperf core gc to analyze things.
- How are pending compactions and pending mutations looking? Again, a tool like sperf core statuslogger help a lot. Rule of thumb though is more than 100 pending compactions or 10000 pending mutations spells trouble.
- How does disk io and cpu usage look? Collect an iostat on your servers. Disk queue length of 1 means your IO isn’t keeping up, this will happen sometimes in a busy but otherwise healthy server, if it’s happening a lot (more than 5%) of the time you’re in for some trouble. If your CPU uses hyperthreading and is busier than 40% of the time a lot of the time (say more than 5% again) that’s probably alos an issue. Use
sperf sysbottle
to analyze your iostat file. - Does my data model generate a lot of GC? If so how can I change it? Validate this by randomly turniing off some of your queries and seeing which ones are the most expensive.
- Is my server well tuned for my hardware? Is CPU or IO pegged? If not how busy are they? Can you add more clients? If not why?
- Should I just add more nodes? Does changing RF have any affect on my reads and write load?
- In cassandra.yaml set
endpoint_snitch: GossipingPropertyFileSnitch
, some prefer PropertyFileSnitch for the ease of pushing out one file. GossipingPropertyFileSnitch is harder to get wrong in my experience. - set dc in cassandra-rackdc.properties. Set to be whatever dc you want that node to be in. Ignore rack until you really need it, 8/10 people that use racks do it wrong the first time, and it’s slightly painful to unwind.
- finish adding all of your nodes.
- if using authentication, set
system_auth
keyspace to use NetworkTopologyStrategy in cqlsh with RF 3 (or == number of replicas if less than 3 per dc) for each datacenter you’ve createdALTER KEYSPACE system_auth WITH REPLICATION= {'class' : 'NetworkTopologyStrategy', 'data_center_name' : 3, 'data_center_name' : 3};
, run repair after changing RF nodetool repair -pr system_auth
on each node in the cluster on the new keyspace.- create your new keyspaces for your app with RF 3 in each dc (much like you did for the
system_auth
step above). nodetool repair -pr whatever_new_keyspace
on each node in the cluster on the new keyspace.- make sure none of the drivers you use to connect to cassnadra are using DowngradingConsistencyRetryPolicy, or using the maligned withUsedHostsPerRemoteDc, especially allowRemoteDCsForLocalConsistencyLevel, as this may cause your driver to send requests to the remote data center before it’s populated with data.
- switch
endpoint_snitch
on each node to GossipingPropertyFileSnitch - set dc in cassandra-rackdc.properties. Set to be whatever dc you want that node to be in. Ignore rack until you really need it, 8/10 people that use racks do it wrong the first time, and it’s slightly painful to unwind.
- bootstrap each node in the new data center.
- if using authentication, set
system_auth
keyspace to use NetworkTopologyStrategy in cqlsh with RF 3 (or == number of replicas if less than 3 per dc) for each datacenter you’ve createdALTER KEYSPACE system_auth WITH REPLICATION= {'class' : 'NetworkTopologyStrategy', 'data_center_name' : 3, 'data_center_name' : 3};
, run repair after changing RF nodetool repair -pr system_auth
on each node in the cluster on the new keyspace.- alter your app keyspaces for your app with RF 3 in each dc (much like you did for the
system_auth
step above), nodetool repair -pr whatever_keyspace
on each node in the cluster on the new keyspace.- let repair jobs continue..that’s it!
- run
nodetool rebuild
on each node in the new dc only, if it dies for some reason, rerunning the command will resume the process. - run
nodetool cleanup
- leave repair jobs running
- run
nodetool rebuild
on each node in the new dc only, if it dies for some reason, rerunning the command will resume the process. - run
nodetool cleanup
on each node - Mega Minimal: website and db, mostly manual on the backend
- Mega Mega: provisioning system, dynamic tuning of systems via ML, automated operations, monitoring a few others I’m leaving out.
- Mega Minimal, they only focused on a single feature, executed well enough that people gave them some positive feedback, but not enough to justify automating everything.
- Mega Mega. I’m not sure anyone who talked about the product saw the same core value, and there were several rewrites and shifts along the way.
- Dep hangs randomly and is slow, which is supposedly network traffic but it happens to everyone I know with tons of bandwidth. Regardless, I’d like an option to supply a timeout and report an error.
- Versions and transitive depency conflicts can be a real breaking issue in Go. So without shading or it’s equivalent two package depending on different versions of a given package can break your build, there are a number or proposals to fix this but we’re not there yet.
- Dep has some goofy ways it resolves transitive dependencies and you may have to add explicit references to them in your Gopkg.toml file. You can see an example here under Updating dependencies – golang/dep.
- Avoid hangs by checking in your dependencies directly into your source repository and just using the dependency tool (dep, godep, glide it doesn’t matter) for downloading dependencies.
- Minimize transitive dependencies by keeping stuff small and using patterns like microservices when your dependency tree conflicts.
- I want to use a package I found on github called jim/awesomeness
- I have to go to ~/go/src and mkdir -p github.com/jim
- cd into that and clone the package.
- When I reference the package in my source file it’ll be literally importing github.com/jim/awesomeness
- myproject/bin for generated executables
- myproject/cmd for command line code
- myproject/pkg for code related to the package
- Find the ideal write size it can make a 10x difference in perf (10k-100k is common).
- Limit threads in flight when writing.
- Use tokenaware unlogged batches if you need to get to your ideal size.
- The entire datacenter is down including your app servers that are supposed to be handling this failover via the LoadBalancingPolicy
- There is something seriously wrong in your data model that is knocking all the nodes off line, if you send this traffic to another data center it will also go off line (assuming you have the bandwidth to get the queries through)
- You’ve created a SPOF in your deployment for Cassandra using SAN or some other infrastructure mistake..don’t do this
- Node A and B are down restarting because a new admin got overzealous.
- A write logging a user’s purchase is destined for A and B in DC1 instead go to their partners in DC2 and the write quietly completes successfully in the remote dc.
- Node A and B and DC1 start responding but do not yet have the write from DC2 propagated back to them.
- The user goes back to read his purchase history which goes to nodes A and B but no does not yet have it.
- Transient failure. Retry is probably faster.
- SLAs are met or the query can just fail at least on the Cassandra side. If you swap at the load balancer level to a distant data center the customer may not have a great experience still, but dependent queries won’t stack up.
- Inter DC bandwidth is largely unaffected.
- Application consistency is maintained.
- Enough operational overhead to handle application servers from multiple data centers talking to a single Cassandra data center
- Enough inter DC bandwidth to support all those app servers talking across the pipe
- High enough SLAs or data centers close enough to hit SLA still,
- Weak consistency needs but a preference for more consistency than what ONE would give you
- Prefer local nodes to remote ones still even though you have enough load, bandwidth and low enough latency to handle all of these queries from any data center.
Picking a Web Microframework
We had a “home grown” framework for a new application we’re working on and the first thing I did was try and rip that out (new project so didn’t have URL and parameter sanitization anyway to do routes, etc).
However, being that the group I was working with has had some bad experiences with “frameworks” I had to settle on something that was lightweight, integrated with Jetty and allowed us to work the way that was comfortable for us as team (also it had to work with Scala).
Microframeworks
The team had shown a lot of disdain for Play (which I had actually quite a lot when I last was leading a JVM based tech stack) and Spring Boot as being too heavy weight, so these were definitely out.
Fortunately, in the JVM world there is a big push back now on heavy web frameworks so meant I had lots of choices for “non frameworks” but could still do some basic security, routing, authentication but not hurt the existing team’s productivity.
There are probably 3 dozen microframeworks to choose from with varying degrees of value but the two that seemed to easiest to start with today were:
My Attempt with Quarkus
Quarkus has a really great getting started story but it’s harder to get started on an existing project with it, it was super trivial to add, and after a couple of days of figuring out the magic incantation I just decided to punt on it. I think because of it’s popularity in the Cloud Native space (which we’re trying to target), the backing of Red Hat, and the pluggable nature of the stack there are a lot of reasons to want this to work. In the end because of the timeline it didn’t make the cut. But it may come back.
My Attempt with Javalin
Javalin despite being a less popular project than Quarkus it is getting some buzz. It also looks like it just slides into the team’s existing Servlet code base. I wanted this to work very badly but stopped before I even started because of this issue so this was out despite being on paper a really execellent framework.
My Attempt with Scalatra
Scalatra has been around for a number of years and is inspired by Sinatra which I used quite a bit in my Ruby years. This took a few minutes to get going just following their standalone directions and then some more to successful convert the routes and account for learning curves with routes.
Some notes:
Conclusion
I think we’re going to stick with Scalatra for the time being as it is a muture framework that works well for our current goals. However, the lack of http/2 support maybe a deal breaker in the medium term.
Getting started with Cassandra: Data modeling in the brief
Cassandra data modeling isn’t really something you can do “in the brief” and is itself a subject that can take years to fully grasp, but this should be a good starting point.
Introduction
Cassandra distributes data around the cluster via the partition key.
CREATE TABLE my_key.my_table_by_postal_code (postal_code text, id uuid, balance float, PRIMARY KEY(postal_code, id));
In the above table the partition key is postal_code
and the clustering column isid
. The partition key will locate the data on the cluster for us. The clustering column allows us multiple rows per partition key so that we can filter how much data we read per partition.
The ‘optimal’ query is one that retrieves data from only one node and not so much data that GC pressure or latency issues result. The following query is breaking that rule and retrieving 2 partitions at once via the IN parameter.
SELECT * FROM my_key.my_table_by_postal_code WHERE postal_code IN ('77002', '77043');
This can be slower than doing two separate queries asynchronously, especially if those partitions are on two different nodes (imagine if there are 1000+ partitions in the IN statement). In summary, the simple rule to stick to is “1 partition per query”.
Partition sizes
A common mistake when data modeling is to jam as much data as possible into a single partition.
Table per query pattern
A common approach to optimize around partition lookup is to create a table per query, and write to all of them on update. The following example has two related tables both to solve two different queries
--query by postal_code
CREATE TABLE my_key.my_table_by_postal_code (postal_code text, id uuid, balance float, PRIMARY KEY(postal_code, id));
SELECT * FROM my_key.my_table_by_postal_code WHERE postal_code = '77002';
--query by id
CREATE TABLE my_key.my_table (id uuid, name text, address text, city text, state text, postal_code text, country text, balance float, PRIMARY KEY(id));
SELECT * FROM my_key.my_table WHERE id = 7895c6ff-008b-4e4c-b0ff-ba4e4e099326;
You can update both tables at once with a logged batch:
BEGIN BATCH
INSERT INTO my_key.my_table (id, name, address, city, state, postal_code, country, balance) VALUES (7895c6ff-008b-4e4c-b0ff-ba4e4e099326, 'Bordeaux', 'Gironde', '33000', 'France', 56.20);
INSERT INTO my_key.my_table_by_postal_code (postal_code, id, balance) VALUES ('33000', 7895c6ff-008b-4e4c-b0ff-ba4e4e099326, 56.20) ;
APPLY BATCH;
Source of truth
A common design pattern is to have one table act as the authoritative one over data, and if for some reason there is a mismatch or conflict in other tables as long as there is one considered “the source of truth” it makes it easy to fix any conflicts later. This is typically the table that would match what we see in typical relational databases and has all the data needed to generate all related views or indexes for different query methods. Taking the prior example, my_table
is the source of truth:
--source of truth table
CREATE TABLE my_key.my_table (id uuid, name text, address text, city text, state text, postal_code text, country text, balance float, PRIMARY KEY(id));
SELECT * FROM my_key.my_table WHERE id = 7895c6ff-008b-4e4c-b0ff-ba4e4e099326;
--based on my_key.my_table and so we can query by postal_code
CREATE TABLE my_key.my_table_by_postal_code (postal_code text, id uuid, balance float, PRIMARY KEY(postal_code, id));
SELECT * FROM my_key.my_table_by_postal_code WHERE postal_code = '77002';
Next we discuss strategies for keeping tables of related in sync.
Materialized views
Materialized views are a feature that ships with Cassandra but is currently considered rather experimental. If you want to use them anyway:
CREATE MATERIALIZED VIEW my_key.my_table_by_postal_code
AS SELECT postal_code text, id uuid, balance float
FROM my_key.my_table
WHERE postal_code IS NOT NULL AND id IS NOT NULL
PRIMARY KEY(postal_code, id));
Materialized views at least run faster than the comparable BATCH insert pattern, but they have a number of bugs and known issues that are still pending fixes.
Secondary indexes
This are the original server side approach to handling different query patterns but it has a large number of downsides:
For just those two reasons I think it’s rare that one can use secondary indexes and expect reasonable performance. However, you can make one by hand and just query that data asynchronously to avoid some of the downsides.
CREATE TABLE my_key.my_table_by_postal_code_2i (postal_code text, id uuid, PRIMARY KEY(postal_code, id));
SELECT * FROM my_key.my_table_by_postal_code_2i WHERE postal_code = '77002';
--retrieve all rows then asynchronously query the resulting ids
SELECT * FROM my_key.my_table WHERE id = ad004ff2-e5cb-4245-94b8-d6acbc22920a;
SELECT * FROM my_key.my_table WHERE id = d30e9c65-17a1-44da-bae0-b7bb742eefd6;
SELECT * FROM my_key.my_table WHERE id = e016ae43-3d4e-4093-b745-8583627eb1fe;
Exercises
Contact List
This is a good basic first use case as one needs to use multiple tables for the same data, but there should not be too many.
requirements
Music Service
Takes the basics from the previous exercise and requires a more involved understanding of the concepts. It will require many tables and some difficult trade-offs on partition sizing. There is no one correct way to do this.
requirements
IoT Analytics
This will require some extensive time series modeling and takes some of the lessons from the Music Service further. The table(s) used will be informed by the query.
requirements
Getting started with Cassandra: Load testing Cassandra in brief
An opinionated guide on the “correct” way to load test Cassandra. I’m aiming to keep this short so I’m going to leave out a lot of the nuance that one would normally get into when talking about load testing cassandra.
If you have no data model in mind
Use cassandra stress since it’s around:
If you have a specific data model in mind
You can use cassandra-stress, but I suspect you’re going to find your data model isn’t supported (collections for example) or that you don’t have the required PHD to make it work the way you want. There are probably 2 dozen options from here you can use to build your load test, some of the more popular ones are gatling, jmeter, and tlp-stress. My personal favorite for this though, write a small simple python or java program that replicates your use case accurately in your own code, using a faker library to generate your data. This takes more time but you tend to have less surprises in production as it will accurately model your code.
Small python script with python driver
import argparse
import uuid
import time
import random
from cassandra.cluster import Cluster
from cassandra.query import BatchStatement
from faker import Faker
parser = argparse.ArgumentParser(description='simple load generator for cassandra')
parser.add_argument('--hosts', default='127.0.0.1',
type=str,
help='comma separated list of hosts to use for contact points')
parser.add_argument('--port', default=9042, type=int, help='port to connect to')
parser.add_argument('--trans', default=1000000, type=int, help='number of transactions')
parser.add_argument('--inflight', default=25, type=int, help='number of operations in flight')
parser.add_argument('--errors', default=-1, type=int, help='number of errors before stopping. default is unlimited')
args = parser.parse_args()
fake = Faker(['en-US'])
hosts = args.hosts.split(",")
cluster = Cluster(hosts, port=args.port)
try:
session = cluster.connect()
print("setup schema");
session.execute("CREATE KEYSPACE IF NOT EXISTS my_key WITH REPLICATION = {'class': 'SimpleStrategy', 'replication_factor': 1}")
session.execute("CREATE TABLE IF NOT EXISTS my_key.my_table (id uuid, name text, address text, state text, zip text, balance int, PRIMARY KEY(id))")
session.execute("CREATE TABLE IF NOT EXISTS my_key.my_table_by_zip (zip text, id uuid, balance bigint, PRIMARY KEY(zip, id))")
print("allow schema to replicate throughout the cluster for 30 seconds")
time.sleep(30)
print("prepare queries")
insert = session.prepare("INSERT INTO my_key.my_table (id, name, address, state, zip, balance) VALUES (?, ?, ?, ?, ?, ?)")
insert_rollup = session.prepare("INSERT INTO my_key.my_table_by_zip (zip, id, balance) VALUES (?, ?, ?)")
row_lookup = session.prepare("SELECT * FROM my_key.my_table WHERE id = ?")
rollup = session.prepare("SELECT sum(balance) FROM my_key.my_table_by_zip WHERE zip = ?")
threads = []
ids = []
error_counter = 0
query = None
params = []
ids = []
def get_id():
items = len(ids)
if items == 0:
## nothing present so return something random
return uuid.uuid4()
if items == 1:
return ids[0]
return ids[random.randint(0, items -1)]
print("starting transactions")
for i in range(args.trans):
chance = random.randint(1, 100)
if chance > 0 and chance < 50:
new_id = uuid.uuid4()
ids.append(new_id)
state = fake.state_abbr()
zip_code = fake.zipcode_in_state(state)
balance = random.randint(1, 50000)
query = BatchStatement()
name = fake.name()
address = fake.address()
bound_insert = insert.bind([new_id, fake.name(), fake.address(), state, zip_code, balance])
query.add(bound_insert)
bound_insert_rollup = insert_rollup.bind([zip_code, new_id, balance])
query.add(bound_insert_rollup)
elif chance > 50 and chance < 75:
query = row_lookup.bind([get_id()])
elif chance > 75:
zip_code = fake.zipcode()
query = rollup.bind([zip_code])
threads.append(session.execute_async(query))
if i % args.inflight == 0:
for t in threads:
try:
t.result() #we don't care about result so toss it
except Exception as e:
print("unexpected exception %s" % e)
if args.errors > 0:
error_counter = error_counter + 1
if error_counter > args.errors:
print("too many errors stopping. Consider raising --errors flag if this happens more quickly than you'd like")
break
threads = []
print("submitted %i of %i transactions" % (i, args.trans))
finally:
cluster.shutdown()
Small java program with latest java driver
package pro.foundev;
import java.lang.RuntimeException;
import java.lang.Thread;
import java.util.Locale;
import java.util.ArrayList;
import java.util.List;
import java.util.function.*;
import java.util.Random;
import java.util.UUID;
import java.util.concurrent.CompletionStage;
import java.net.InetSocketAddress;
import com.datastax.oss.driver.api.core.CqlSession;
import com.datastax.oss.driver.api.core.CqlSessionBuilder;
import com.datastax.oss.driver.api.core.cql.*;
import com.github.javafaker.Faker;
public class App
{
public static void main( String[] args )
{
List<String> hosts = new ArrayList<>();
hosts.add("127.0.0.1");
if (args.length > 0){
hosts = new ArrayList<>();
String rawHosts = args[0];
for (String host: rawHosts.split(",")){
hosts.add(host.trim());
}
}
int port = 9042;
if (args.length > 1){
port = Integer.valueOf(args[1]);
}
long trans = 1000000;
if (args.length > 2){
trans = Long.valueOf(args[2]);
}
int inFlight = 25;
if (args.length > 3){
inFlight = Integer.valueOf(args[3]);
}
int maxErrors = -1;
if (args.length > 4){
maxErrors = Integer.valueOf(args[4]);
}
CqlSessionBuilder builder = CqlSession.builder();
for (String host: hosts){
builder = builder.addContactPoint(new InetSocketAddress(host, port));
}
builder = builder.withLocalDatacenter("datacenter1");
try(final CqlSession session = builder.build()){
System.out.println("setup schema");
session.execute("CREATE KEYSPACE IF NOT EXISTS my_key WITH REPLICATION = {'class': 'SimpleStrategy', 'replication_factor': 1}");
session.execute("CREATE TABLE IF NOT EXISTS my_key.my_table (id uuid, name text, address text, state text, zip text, balance int, PRIMARY KEY(id))");
session.execute("CREATE TABLE IF NOT EXISTS my_key.my_table_by_zip (zip text, id uuid, balance bigint, PRIMARY KEY(zip, id))");
System.out.println("allow schema to replicate throughout the cluster for 30 seconds");
try{
Thread.sleep(30000);
}catch(Exception ex){
throw new RuntimeException(ex);
}
System.out.println("prepare queries");
final PreparedStatement insert = session.prepare("INSERT INTO my_key.my_table (id, name, address, state, zip, balance) VALUES (?, ?, ?, ?, ?, ?)");
final PreparedStatement insertRollup = session.prepare("INSERT INTO my_key.my_table_by_zip (zip, id, balance) VALUES (?, ?, ?)");
final PreparedStatement rowLookup = session.prepare("SELECT * FROM my_key.my_table WHERE id = ?");
final PreparedStatement rollup = session.prepare("SELECT sum(balance) FROM my_key.my_table_by_zip WHERE zip = ?");
final List<UUID> ids = new ArrayList<>();
final Random rnd = new Random();
final Locale us = new Locale("en-US");
final Faker faker = new Faker(us);
final Supplier<UUID> getId = ()-> {
if (ids.size() == 0){
//return random uuid will be record not found
return UUID.randomUUID();
}
if (ids.size() == 1){
return ids.get(0);
}
final int itemIndex = rnd.nextInt(ids.size()-1);
return ids.get(itemIndex);
};
final Supplier<Statement<?>> getOp = ()-> {
int chance = rnd.nextInt(100);
if (chance > 0 && chance < 50){
final String state = faker.address().stateAbbr();
final String zip = faker.address().zipCodeByState(state);
final UUID newId = UUID.randomUUID();
final int balance = rnd.nextInt();
ids.add(newId);
return BatchStatement.builder(BatchType.LOGGED)
.addStatement(insert.bind(newId,
faker.name().fullName(),
faker.address().streetAddress(),
state,
zip,
balance))
.addStatement(insertRollup.bind(zip, newId, Long.valueOf(balance)))
.build();
} else if (chance > 50 && chance < 75){
return rowLookup.bind(getId.get());
}
final String state = faker.address().stateAbbr();
final String zip = faker.address().zipCodeByState(state);
return rollup.bind(zip);
};
System.out.println("start transactions");
List<CompletionStage<AsyncResultSet>> futures = new ArrayList<>();
int errorCounter = 0;
for (int i = 0; i < trans; i++){
//this is an uncessary hack to port old code and cap transactions in flight
if ( i % inFlight == 0){
for (CompletionStage<AsyncResultSet> future: futures){
try{
future.thenRun(()->{});
}catch(Exception ex){
if (maxErrors > 0){
if (errorCounter > maxErrors){
System.out.println("too many errors therefore stopping.");
break;
}
errorCounter += 1;
}
}
}
futures = new ArrayList<>();
System.out.println("submitted " + Integer.toString(i) + " of " + Long.toString(trans) + " transactions");
}
Statement<?> query = getOp.get();
futures.add(session.executeAsync(query));
}
}
}
}
How to measure performance
The above scripts and examples are not ideal for several points but if you throw enough clients at the cluster at the same time those problems should balance out (namely the pseudo random distributions aren’t ideal). But let’s assume you’ve worked these points out or gone with a gatling or other such tool what sort of issues should you look for now:
Summary
There are dozens of other good measurements to look at when trying to monitor load on your server and observe what your server can handle, but this is supposed to be a quick and dirty guide. So try these out for starters and ask yourself the following questions:
Getting started with Cassandra: Setting up a Multi-DC environment
This is a quick and dirty opinionated guide to setting up a Cassandra cluster with multiple data centers.
A new cluster
An existing cluster
This is harder and involves more work and more options, but I’m going to discuss the way that gets you into the least amount of trouble operationally.
enjoy new data center
how to get data to new dc
Repair approach
Best done with if your repair jobs can’t be missed or stopped, either because you have a process like opscenter or repear running repairs. It also has the advantage of being very easy, and if you’ve already automated repair you’re basically done.
Rebuild approach
Faster less resource intensive, and if you have enough time to complete it while repair is stopped. Rebuild is easier to ‘resume’ than repair in many ways, so this has a number of advantages.
YOLO rebuild with repair
This will probably overstream it’s share of data and honestly a lot of folks do this for some reason in practice:
Cloud strategies
There are a few valid approaches to this and none of them are wrong IMO.
region == DC, rack == AZ
Will need to get into racks and a lot of people get this wrong and imbalance the racks, but you get the advantage of more intelligent failure modes, with racks mapping to AZs.
AZ..regardless of region == DC
This allows things to be balanced easily, but you have no good option for racks then. However, some people think racks are overated, and I’d say a majority of clusters run with one rack.
MVP how minimal
MVPs or Minimum Viable Products are pretty contentious ideas for something seemingly simple. Depending on background and where pepole are coming from experience wise those terms carry radically different ideas. In recent history I’ve seen up close two extreme constrasting examples of MVP:
Feedback
If we’re evaluating which approach gives us more feedback, Mega Minimal MVP is gonna win hands down here. Some will counter they don’t want to give people a bad impression with a limited product and that’s fair, but it’s better than no impression (the dreaded never shipped MVP). The Mega Mega MVP I referenced took months to demo. only had one of those checkboxes setup and wasn’t ever demod again. So we can categorical say that failed at getting any feedback.
Whereas the Mega Minimal MVP, got enough feedback and users for the founders to realize that wasn’t a business for them. Better than after hiring a huge team and sinking a million plus into dev efforts for sure. Not the happy ending I’m sure you all were expecting, but I view that as mission accomplished.
Core Value
Advantage Mega Minimal again
What about entrants into a crowded field
Well that is harder and the MVP tends to be less minimal, because the baseline expectations are just much higher. I still lean towards Mega Minimal having a better chance at getting users, since there is a non zero chance the Mega Mega MVP will never get finished. I still think the exercise in focusing on core value that makes your product not a me too, and even considering how you can find a niche in a crowded field instead of just being “better”, and your MVP can be that niche differentiator.
Internal users
Sometimes a good middle ground is considering getting lots of internal users if you’re really worried about bad experiences. This has it’s it’s definite downsides however, and you may not get diverse enough opinions. But it does give you some feedback while saving some face or bad experiences. I often think of the example of EC2 that was heavily used by Amazon, before being released to the world. That was a luxury Amazon had, where their customer base and their user base happened to be very similar, and they had bigger scale needs than any of their early customers, so the early internal feedback loop was a very strong signal.
Summary
In the end however you want to approach MVPs is up to you, and if you find success with a meatier MVP than I have please don’t let me push you away from what works. But if you are having trouble shipping and are getting pushed all the time to add one more feature to that MVP before releasing it, consider stepping back and asking is this really core value for the product? Do you already have your core value? if so, consider just releasing it.
Surprise Go is ok for me now
I’m surprised to say this. I am ok using Go now. It’s not my style, but I can build almost anything I want to with it, and the tooling around it continues to improve.
About seven months ago, I wrote about all the things I didn’t care for in Go and now I either no longer am so bothered by it, or things have improved.
Go Modules so far is a massive improvement over Dep and Glide for dependency management. It’s easy to set up, performant and eliminates the GOPATH silliness. I no longer have to check-in the vendor directory to speed up builds. Lesson use Go Modules.
I pretty much stopped using channels for everything but shutdown signals, which fits my preferences pretty well. I use mutex and semaphores for my multithreaded code and feel no guilt about it. The strategy of avoiding channels cut out a lot of pain for me, and with the excellent race detector, I feel comfortable writing multithreaded in Go now. The lesson, don’t use channels much.
Lack of generics still sometimes sucks, but I usually implement some crappy casting with dynamic types if I need that. I’ve made my peace by writing more code and am no longer so hung up. Lesson relax.
With the Error handling in Go, I’m still struggling. I thought about using one of the error Wrap() libraries, but an official one is in the draft spec now, so I’ll wait on that. I now tend to have less nesting of functions. As a result, this probably means more extended functions than I like, but my code looks more “normal” now. I am ok with trading off my ideal code; that may not be as ideal as I think it is if it makes my code more widely accepted. Lesson relax more.
I see the main virtue of Go now that it is prevalent in the infrastructure space where I am, and so it’s becoming the common tongue (essentially replacing Python for those sorts of tasks). For this, honestly, it’s about right. It’s easy to rip out command-line tools and deploy binaries for every platform with no runtime install.
The community’s conservative attitude I sort of view as a feature now, in that there isn’t a bunch of different popular options, and there is no arguing over what file format rules to use. The “one format to rule them all” drove me up the wall initially, but I appreciate how much less time I spend on these things now.
So now I suspect Go will be my “last” programming language. It’s not the one I would have chosen, but where I am in my career, where most of my dev work is automation and tooling, it fits the bill pretty well.
Also equally important, most people working with me didn’t have full-time careers as developers or spend their time reading “Domain Driven Design” (fantastic book). Therefore, adding in a bunch of nuanced stuff that maybe technically optimal for some situations, which also assumes the reader grasps all of the said nuances, isn’t a good tradeoff for anyone.
So I think I get it now. I’ll never be a cheerleader for the language, but it solves my problems well enough.
Lessons from a year of Golang
I’m hoping to share in a non-negative way help others avoid the pitfalls I ran into with my most recent work building infrastructure software on top of a Kubernetes using Go, it sounded like an awesome job at first but I ran into a lot of problems getting productive.
This isn’t meant to evaluate if you should pick up Go or tell you what you should think of it, this is strictly meant to help people out that are new to the language but experienced in Java, Python, Ruby, C#, etc and have read some basic Go getting started guide.
Dependency management
This is probably the feature most frequently talked about by newcomers to Go and with some justification, as dependency management in Go has been a rapidly shifting area that’s nothing like what experienced Java, C#, Ruby or Python developers are used to.
Today, the default tool is Dep all other tools I’ve used such as Glide or Godep are deprecated in favor of Dep, and while Dep has advanced rapidly there are some problems you’ll eventually run into (or I did):
My advice
GOPATH
Something that takes some adjustment is you check out all your source code in one directory with one path (by default ~/go/src ) and include the path to the source tree to where you check out. Example:
A better guide to GOPATH and packages is here.
My advice
Don’t fight it, it’s actually not so bad once you embrace it.
Code structure
This is a hot topic and there are a few standards for the right way to structure you code from projects that do “file per class” to giant files with general concept names (think like types.go and net.go). Also if you’re used to using a lot of sub package you’re gonna to have issues with not being able to compile if for example you have two sub packages reference one another.
My Advice
In the end I was reasonably ok with something like the following:
Now whatever you do is fine, this was just a common idiom I saw, but it wasn’t remotely all projects. I also had some luck with just jamming everything into the top level of the package and keeping packages small (and making new packages for common code that is used in several places in the code base). If I ever return to using Go for any reason I will probably just jam everything into the top level directory.
Debugging
No debugger! There are some projects attempting to add one but Rob Pike finds them a crutch.
My Advice
Lots of unit tests and print statements.
No generics
Sorta self explanatory and it causes you a lot of pain when you’re used to reaching for these.
My advice
Look at the code generation support which uses pragmas, this is not exactly the same as having generics but if you have some code that has a lot of boiler plate without them this is a valid alternative. See this official Go Blog post for more details.
If you don’t want to use generation you really only have reflection left as a valid tool, which comes with all of it’s lack of speed and type safety.
Cross compiling
If you have certain features or dependencies you may find you cannot take advantage of one of Go’s better features cross compilation.
I ran into this when using the confluent-go/kafka library which depends on the C librdkafka library. It basically meant I had to do all my development in a Linux VM because almost all our packages relied on this.
My Advice
Avoid C dependencies at all costs.
Error handling
Go error handling is not exception base but return based, and it’s got a lot of common idioms around it:
myValue, err := doThing()
if err != nil {
return -1, fmt.Errorf(“unable to doThing %v”, err)
}
Needless to say this can get very wordy when dealing with deeply nested exceptions or when you’re interacting a lot with external systems. It is definitely a mind shift if you’re used to the throwing exceptions wherever and have one single place to catch all exceptions where they’re handled appropriately.
My Advice
I’ll be honest I never totally made my peace with this. I had good training from experienced opensource contributors to major Go projects, read all the right blog posts, definitely felt like I’d heard enough from the community on why the current state of Go error handling was great in their opinions, but the lack of stack traces was a deal breaker for me.
On the positive side, I found Dave Cheney’s advice on error handling to be the most practical and he wrote a package containing a lot of that advice, I found it invaluable as it provided those stack traces I missed.
Summary
A lot of people really love Go and are very productive with it, I just was never one of those people and that’s ok. However, I think the advice in this post is reasonably sound and uncontroversial. So, if you find yourself needing to write some code in Go, give this guide a quick perusal. Hope it helps.
Cassandra: Batch Loading Without the Batch — The Nuanced Edition
My previous post on this subject has proven extraordinarily popular and I get commentary on it all the time, most of it quite good. It has however, gotten a decent number of comments from people quibbling with the nuance of the post and pointing out it’s failings, which is fair because I didn’t explicitly spell this out as a “framework of thinking” blog post or a series of principles to consider. This is the tension between making something approachable and understandable to the new user but still technically correct for the advanced one. Because of where Cassandra was at the time and the user base I was encountering day to day, I took the approach of simplification for the sake of understanding.
However, now I think is a good time to write this up with all the complexity and detail of a production implementation and the tradeoffs to consider.
TLDR
Details on all this below.
You cannot escape physics {#23cb}
Ok it’s not really physics, but it’s a good word to use to get people to understand you have to consider your hard constraints and that wishing them away or pretending they’re not there will not save you from their factual nature.
So now lets talk about some of the constraints that will influence your optimum throughput strategy.
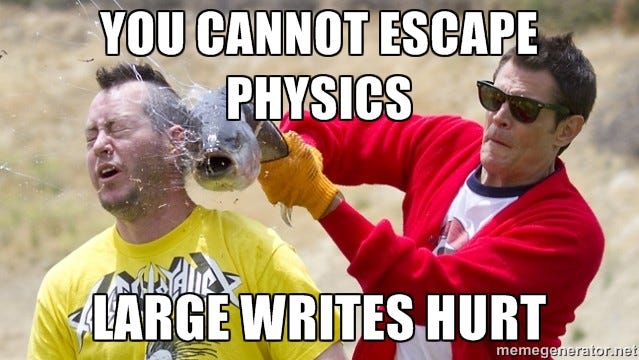
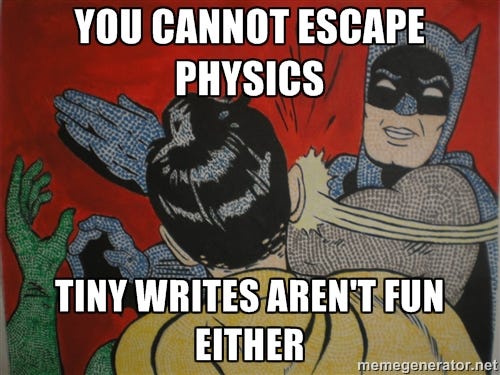
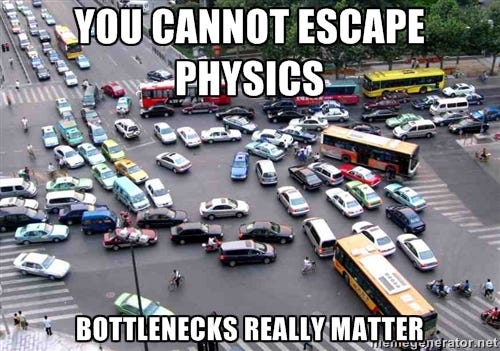
Can your network handle 100 of your 100mb writes? Can your disk? Can your memory? Can your client? Pay attention to whatever gets pegged during load and that should drive the size of your writes and your strategy for using unlogged batches or not.
RECORD COUNT BARELY MATTERS {#1670}
I’m dovetailing here with the previous statement a bit and starting with something inflammatory to get your attention. But this is often the metric I see people use and it’s at best accidentally accurate.
I’ve had some very smart people tell me “100 row unlogged batches that are token aware are the best”. Are those 100 rows all 1GB a piece? or are they all 1 byte a piece?
Your blog was wrong! {#0988}
I had many people smarter than I tell me my “post was wrong” because they were getting awesome performance by ignoring it. None of this is surprising. In some of those cases they were still missing the key component of what matters, in other cases rightly poking holes in the lack of nuance in my previous blog post on this subject (I never clarified it was intentionally simplistic).
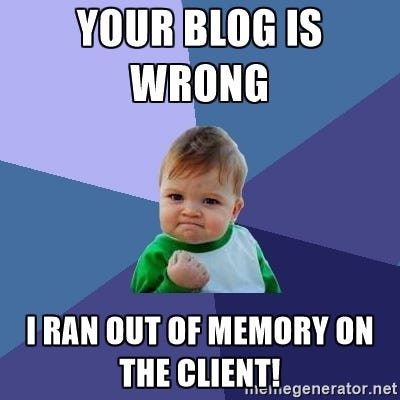
I intentionally left out code to limit threads in flight. Many people new to Cassandra are often new to distributed programming and consequentially multi-threaded programming. I was in this world myself for a long time. Your database, your web framework and all of your client code do everything they can to hide threads from you and often will give you nice fat thread safe objects to work with. Not everyone in computer science has the same background and experience, and the folks in traditional batch jobs programming which is common in insurance and finance where I started do not have the same experience as folks working with distributed real time systems.
In retrospect I think this was a mistake and I ran into a large number of users that did copy and paste my example code from the previous blog post and ran into issues with clients exhausting resources. So here is an updated example using the DataStax Java driver that will allow you to adjust thread levels easily and tweak the amount of threads in flight:
version 2.1–3.0:
Using classic manual manipulation of futures {#3a17}
This is with a fixed thread pool and callbacks
Either approach works as well as the other. Your preference should fit your background.
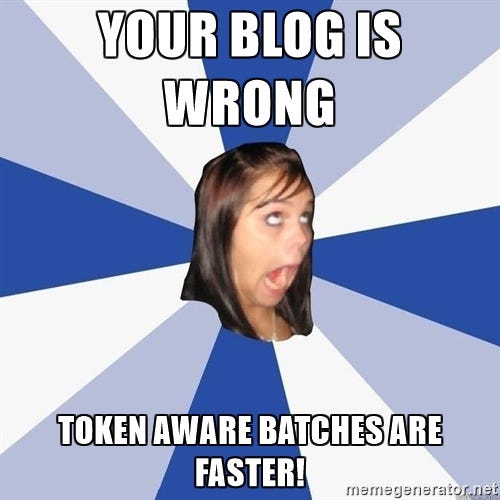
Anyway it is advanced code and I did not think it appropriate for a beginner level blog post. I will later dedicate an entire blog post just to this.
Conclusion {#12a2}
I hope you found the additional nuance useful and maybe this can help some of you push even faster once all of these tradeoffs are taken into account. But I hope you take away my primary lesson:
Distributed databases often require you to apply distributed principles to get the best experience out of them. Doing things the way we’re used to may lead to surprising results.
CASSANDRA LOCAL_QUORUM SHOULD STAY LOCAL
A couple of times a week I get a question where someone wants to know how to “failover” to a remote DC in the driver if the local Cassandra DC fails or even if there is only a couple of nodes in the local data center that are down.
Frequently the customer is using LOCAL_QUORUM or LOCAL_ONE at our suggestion to pin all reads and writes to the local data center often times at their request to help with p99 latencies. But now they want the driver to “do the right thing” and start using replicas in another data center when there is a local outage.
Here is how (but you DO NOT WANT TO DO THIS)
However you may end up with more than you bargained for.
failed
Here is why you DO NOT WANT TO DO THIS
Intended case is stupidly rare
The common case I hear is “my local Cassandra DC has failed” Ok stop..so your app servers in the SAME DATACENTER are up but your Cassandra nodes are all down.
I’ve got news for you, if all your local Cassandra nodes are down one of the following has happened:
So on the off hand that by dumb luck or just neglect you hit this scenario, then sure go for it, but it’s not going to be free (I cover this later on so keep reading).
Common failure cases aren’t helped {#commonfailurecasesaren’thelped}
A badly thought out and unproven data model is the most common reason outside of SAN I see widespread issues with a cluster or data center, failover at a datacenter level will not help you in either case here.
Failure is often transient
More often you may have a brief problem where a couple of nodes are having a problem. Say one expensive query happened right before, or you restarted a node, or you made a simple configuration change. Do you want your queries bleeding out to the other data center because of a very short term hiccup? Instead you could have had just retried the query one more time, the local nodes would have responded before the remote data center had time to receive the query.
TLDR More often than not the correct behavior is just to retry in the local data center.
Application SLAs are not considered
Electrons only move so fast, and if you have 300 ms latency between your London and Texas data centers, while your application has a 100ms SLA for all queries, you’re still basically “down”.
Available bandwidth is not considered
Now lets say the default latency is fine, how fat is that pipe? Often on long links companies go cheap and stay in the sub 100mb range. This can barely keep up with replication traffic from Cassandra for most use cases let alone shifting all queries over. That 300ms latency will soon climb to 1 second or eventually just out and out failure as the pipe totally jams full. So you’re still down.
Operational overhead of the “failed to” data center are not considered {#operationaloverheadofthe“failedto”datacenterarenotconsidered}
I’ve mentioned this in passing a few times, but lets say you have fast enough pipes, can your secondary data center handle not only it’s local traffic but also the new ‘failover’ traffic coming in? If you’ve not allowed for that operational overhead you maybe taking down all data centers one at a time until there is none remaining.
Intended consistency is not met
Now lets say you have operational overhead, latency and bandwidth overhead to connect to that remote data center HUZZAH RIGHT!
Say your app is using LOCAL_QUORUM. This implies something about your consistency needs AKA you need your model to be relatively consistent. Now if you recall above I’ve mentioned that most failures in practice are transient and not permanent.
So what happens in the following scenario?
Now all of this happened without an error and the application server had no idea it should retry. I’ve now defeated the point of LOCAL_QUORUM and I’m effectively using consistency level of TWO without realizing it
What should you do?
Same thing you were before. Use technology like Eureka, F5, whatever customer solution you have or want to use.
I think there is some confusion in terminology here, Cassandra data centers are boundaries to lock in queries and by extension provide some consistency guarantee (some of you will be confused by this statement but it’s a blog post unto itself so save that for another time) and potentially to pin some administrative operations to a set of nodes. However, new users expect the Cassandra data center to be a driver level failover zone.
If you need queries to go beyond a data center then use the classic QUORUM consistency level or something like SERIAL, it’s globally honest and provides consistency at a cluster level in exchange for latency hits in the process, but you’ll get the right answer more often than not that way (SERIAL especially as it takes into account race conditions)
So lets step through the above scenarios:
One scenario that still isn’t handled is having adequate capacity in the downstream data centers. But it’s still something you have to think about either way. On the whole uptime and customer experience are way better with failing over using a dedicated load balancer than trying to do Multidc failover with the Cassandra client.
So is there any case I can use allowRemoteHosts?
Of course but you have to have the following conditions:
Basically the use of this feature is EXTRAORDINARILY minimal and at best only gives you a minor benefit over just using a consistency level of TWO.
Connection to Oracle From Spark
For some silly reason there is a has been a fair amount of difficulty in reading and writing to Oracle from Spark when using DataFrames.
SPARK-10648 — Spark-SQL JDBC fails to set a default precision and scale when they are not defined in an oracle schema.
This issue manifests itself when you have numbers in your schema with no precision or scale and attempt to read. They added the following dialect here
This fixes the issue for 1.4.2, 1.5.3 and 1.6.0 (and DataStax Enterprise 4.8.3). However recently there is another issue.
SPARK-12941 — Spark-SQL JDBC Oracle dialect fails to map string datatypes to Oracle VARCHAR datatype
And this lead me to this SO issue. Unfortunately, one of the answers has a fix they claim only works for 1.5.x however, I had no issue porting it to 1.4.1. The solution in Java looked something like the following, which is just a Scala port of the SO answer above ( This is not under warranty and it may destroy your server, but this should allow you to write to Oracle.)
In the future keep an eye out for more official support in SPARK-12941 and then you can ever forget the hacky workaround above.
subscribe via RSS